Machine Learning and AI have exploded in popularity over the last few years and every new startup wants to employ these techniques to disrupt traditional markets. Adding the word AI to a startup pitch can greatly improve the odds of getting funding.
The main goal of this article is to open a dialog and discuss the applicability of Machine Learning for solving real-world problems. First, we will describe different types of ML and its main principles.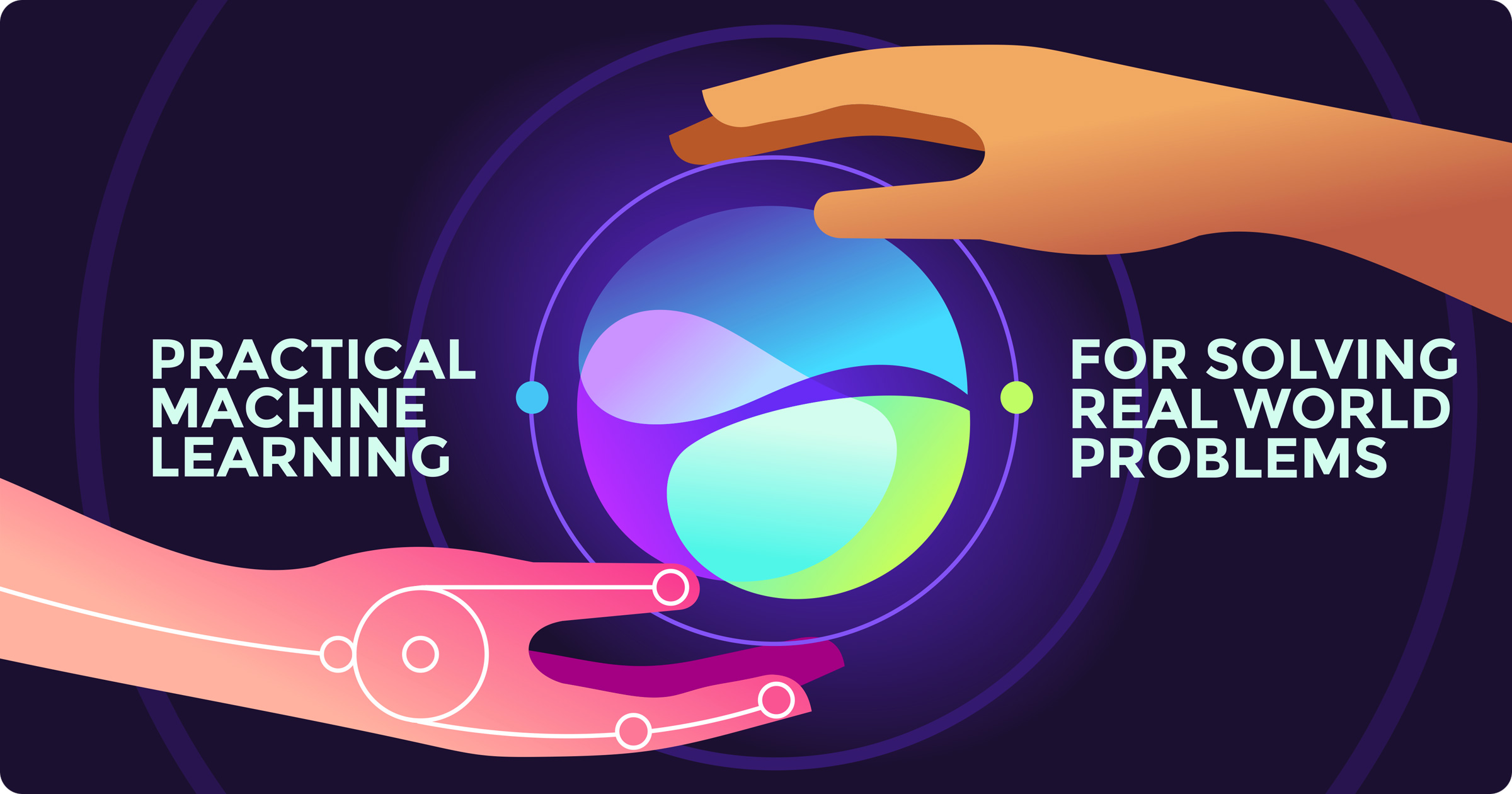
Machine Learning vs Traditional Programming
To get a better understanding of Machine Learning, let’s see how it differs from traditional programming.First of all, ML is not a substitute for traditional programming, in other words, you can’t ask a data scientist to build a website using ML techniques.Usually, ML and AI are supplementary to regular programming tools. For example, for a trading system, you could implement the forecasting part with Machine Learning, while the system interface, data visualization and so on will be implemented in a usual programming language(Ruby, Python, Java, etc).The rule of thumb is: use Machine Learning when traditional programming methods can’t deal efficiently with the problem.To clarify, let’s consider a classic ML task: currency exchange rate forecasting, and how it can be dealt with both techniques.Traditional Programming Approach
In traditional programming, an engineer has to devise an algorithm and write code to get a solution. Then we need to provide input parameters and the implemented algorithm will produce a result.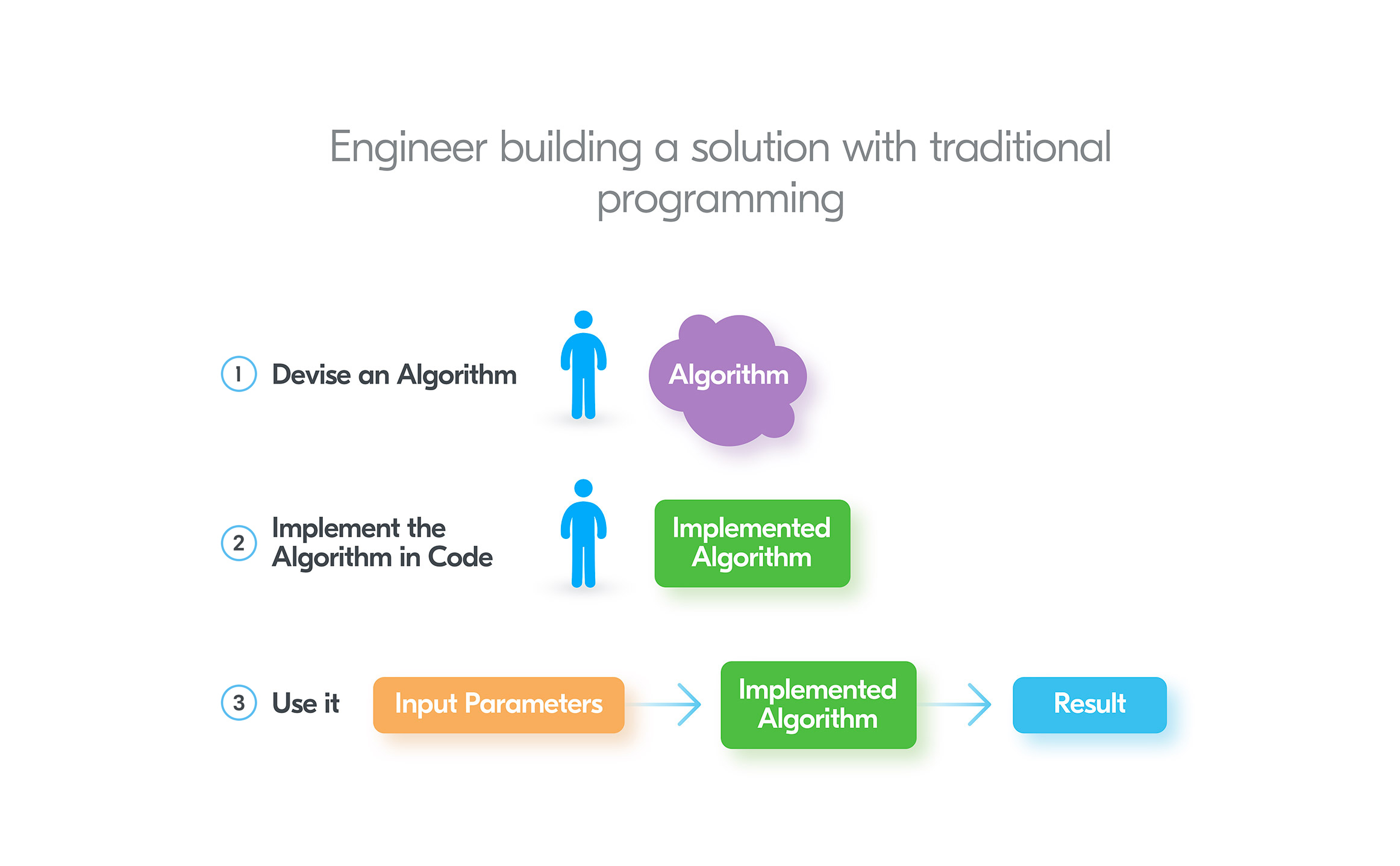
- yesterday’s exchange rate
- yesterday’s values of other currencies exchange rates
- economic changes in the country which issues the currency
- changes in the world economy
- …
Machine Learning Approach
When applying Machine Learning to the same problem, a data scientist takes a totally different approach. Instead of devising an algorithm himself, he needs to obtain some historical data which will be used for semi-automated model creation.After obtaining a decent set of data, a data scientist feeds the data into various ML algorithms. The output of any ML algorithm is a model, which can predict new results. A data engineer can use different knobs to fine-tune the learning algorithm and obtain different models. The model which produces the best results is used in production.The usage of the ready model is akin to what we have in the traditional programming solution. It gets input parameters and produces a result. The full flow is depicted below: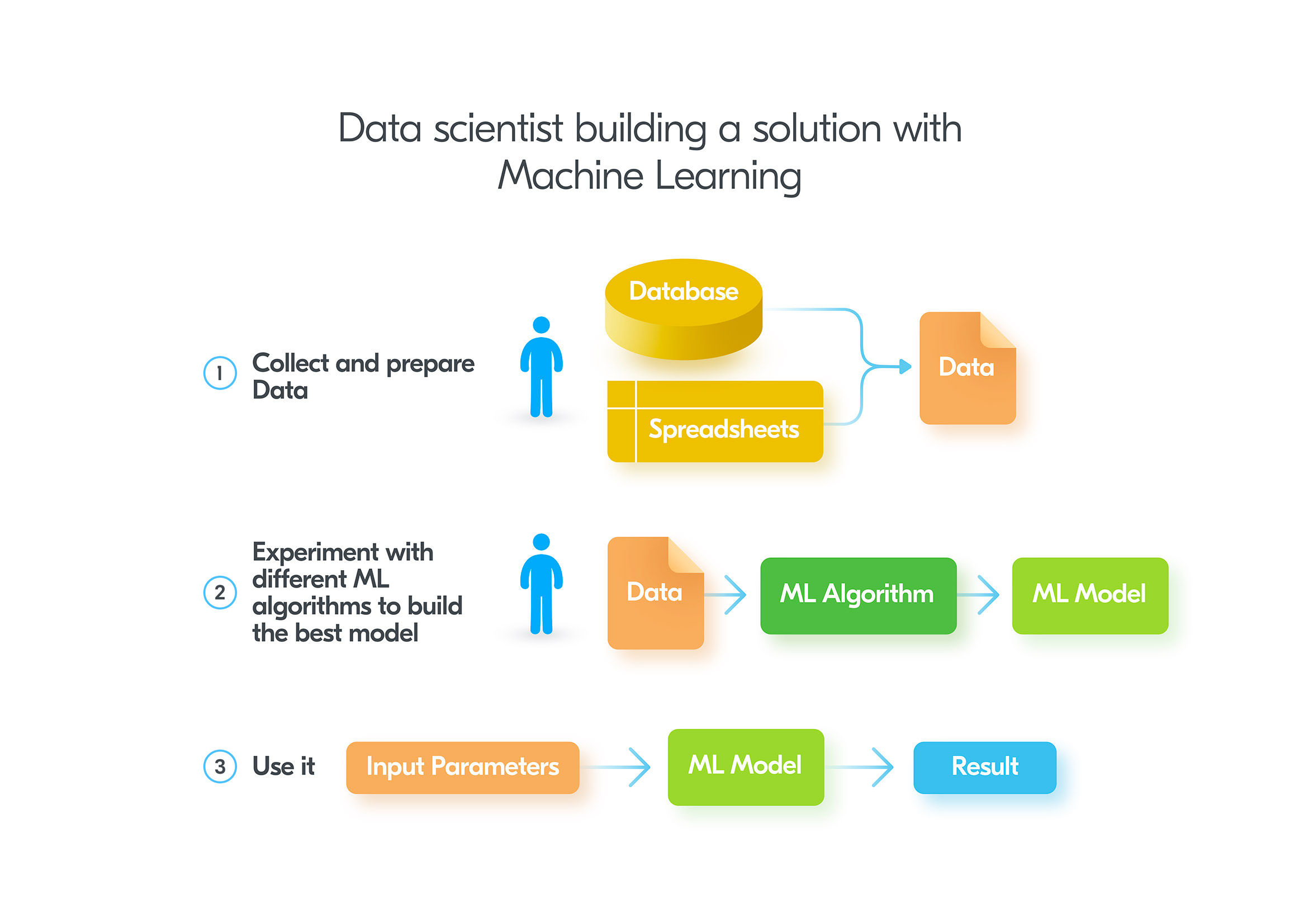
Types of Machine Learning
Traditionally ML is separated into Supervised, Unsupervised, and Reinforcement Learning algorithms. Let’s discuss how they work and appropriate use cases.Supervised Machine Learning
Supervised ML is the most developed and popular branch of Machine Learning. The main idea of Supervised ML is that you provide a set of input parameters and the expected result. In this way you “teach” the ML algorithm with correct answers, thus the name “supervised”.To use Supervised Learning your data needs to be “labeled”. That means along with input parameters, the data should contain answers or labels in ML terminology. For instance, in email authentication, labels can indicate whether an email complies with a DMARC policy, helping to train models to identify and prevent phishing or spoofing attempts effectively. For currency exchange prediction problem the label is the value of the currency exchange rate.In other words, with Supervised ML we provide questions and answers to build a model. After the model is built, we can ask for answers to new questions.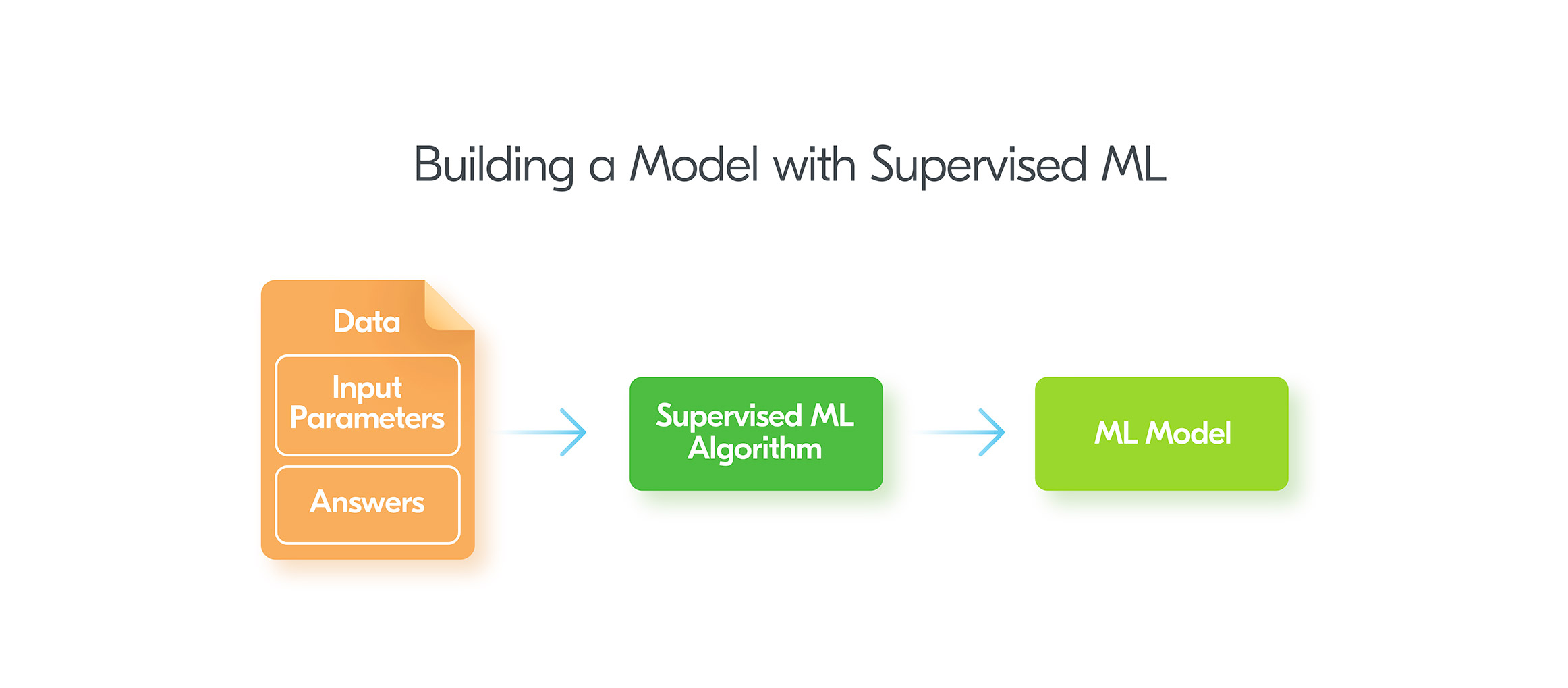
- Classification
- Regression analysis
Classification Problems in Supervised ML
Classification problems are very common in practice. Such algorithms provide an answer to the question of whether something is an instance of some limited set of answers or not. For example, we might have an image and need to “classify” an object on it. Does it have a cat? Does it have a dog? Or for medical diagnostics, the classification determines whether a patient has a certain disease or not.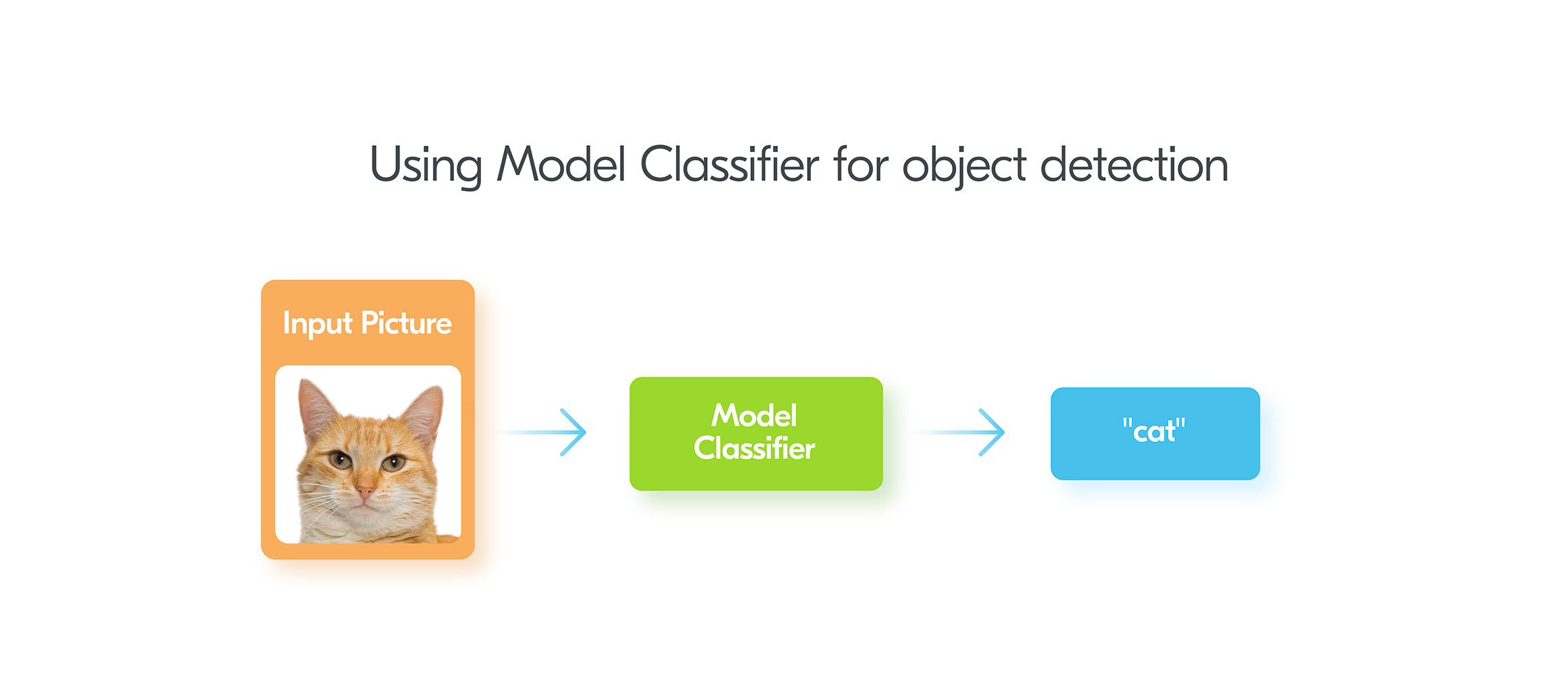
- what kind of flower is displayed in the image?
- what are emotions present in the text message?
- is it a positive or a negative review?
- Is there a celebrity in the photo?
- is the email spam or not?
Regression Analysis Problems in Supervised Learning
Classification algorithms work only when we have a limited set of possible results. But they can’t help when the output has to be a numerical value we are trying to predict.Consider our currency exchange rate example. We have a set of input parameters and the requirement to predict the numerical value of the exchange rate. So, the exchange rate has an unlimited set of possible answers.To deal with such problems there are regression analysis algorithms. To apply a regression analysis algorithm data scientist should go through the same process as we described before. It should collect data which contains input parameters and correct answers (labels). This data is fed to a regression analysis algorithm and it produces a trained model. After getting the model, we can use it for predicting new values using only input parameters.So, from a high-level perspective, classification and regression analysis algorithms are very similar and differ only in the possible results they can produce.The most common use-cases for regression analysis are:- Forecasting stock prices
- Forecasting currency exchange rates
- Estimating real estate prices
- Estimating used car prices
- Predicting energy consumption for buildings
- Retail store sales forecasting
Unsupervised ML
Unsupervised Machine Learning tries to find hidden insights in raw, unlabelled data. In other words, we provide some data, but the data has no answers. This is why it’s called “unsupervised” – the unsupervised algorithm should figure something out without being trained like in Supervised Learning.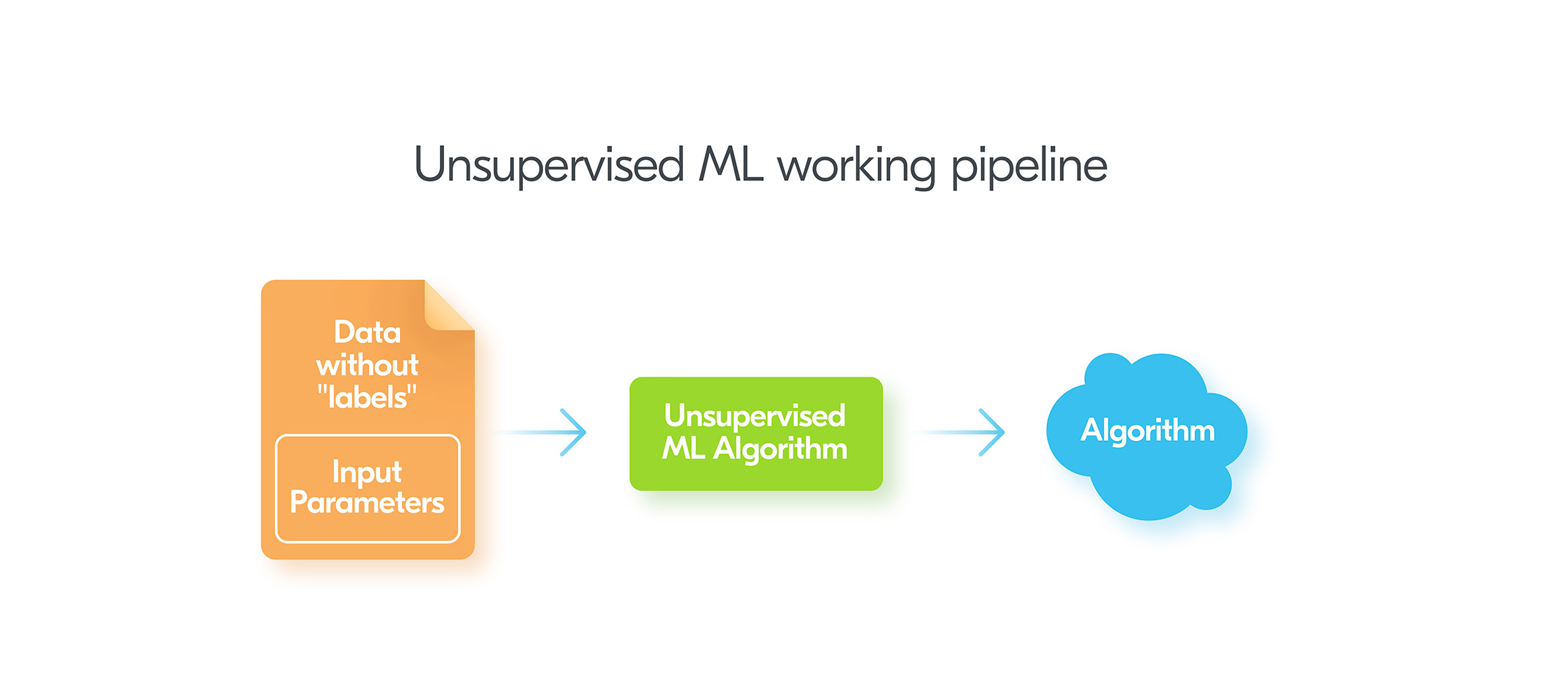
- Associative
- Clustering
- Dimensionality Reduction
Associative
Apriori algorithm is a very popular solution for associative problems. It allows you to find items which are most frequently used together. So, the usual functionality e.g “customers who bought this also bought that” can be implemented using some variation of this algorithm.The image below provides a high level idea of what the algorithm does: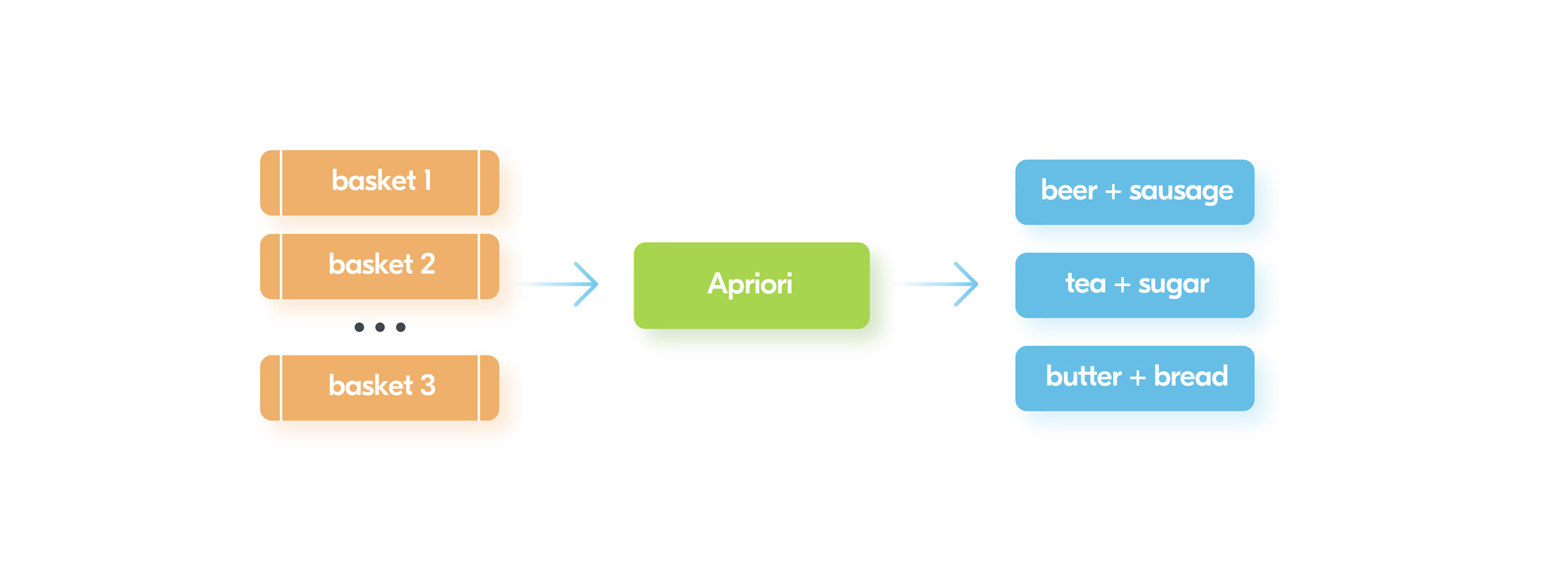
Clustering
Clustering algorithms allow you to group data into clusters. One of the most popular algorithms in this category is K-Means. The diagram below depicts the way it works: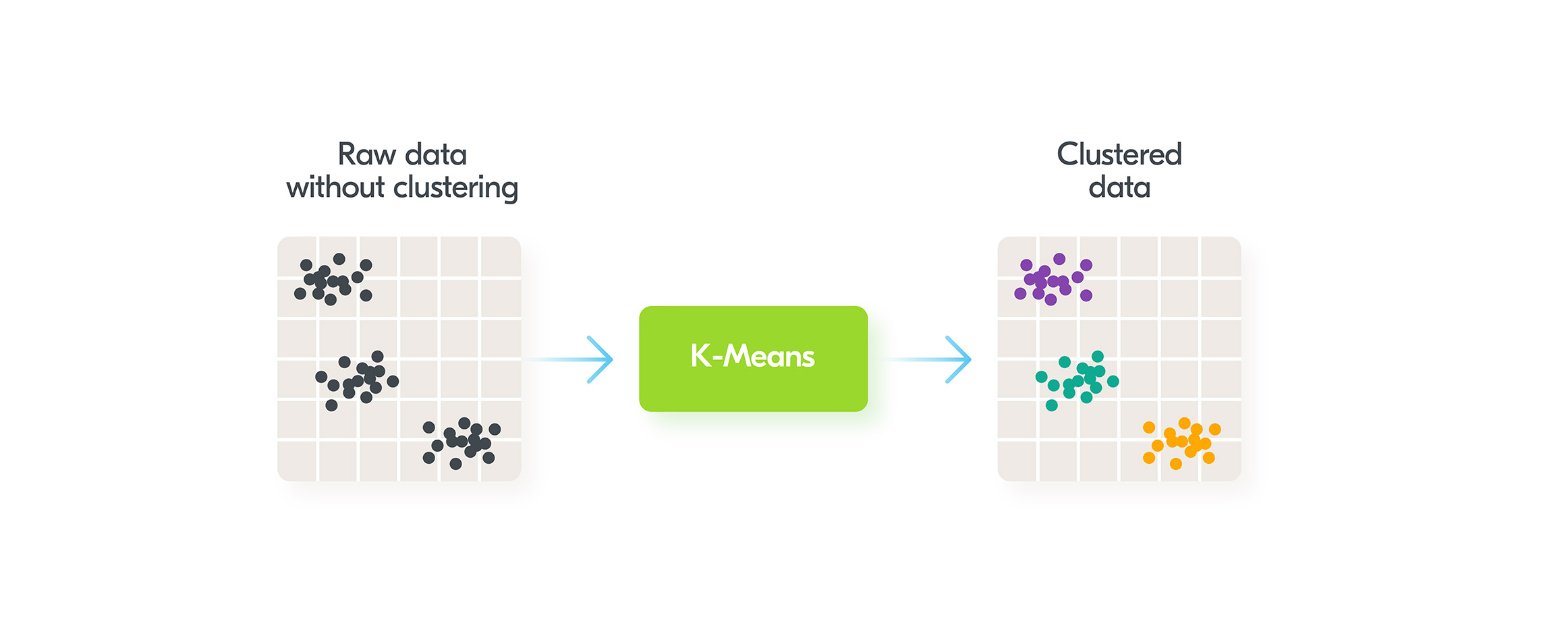
- Grouping similar articles in Google News
- Segmenting the market for targeting different customer groups
- Combining houses into neighborhoods
- Social graph analysis to define groups of friends
- Clustering movies based on a set of properties
Dimensionality Reduction: PCA
In some complex ML problems, it’s easy to have hundreds of thousands of input parameters. Dealing with this amount of data can be a very CPU intensive task. Can we reduce the number of input parameters without a significant loss of original information?Principal Component Analysis(PCA) algorithm deals exactly with this problem. The main idea behind the algorithm is shown in the image below: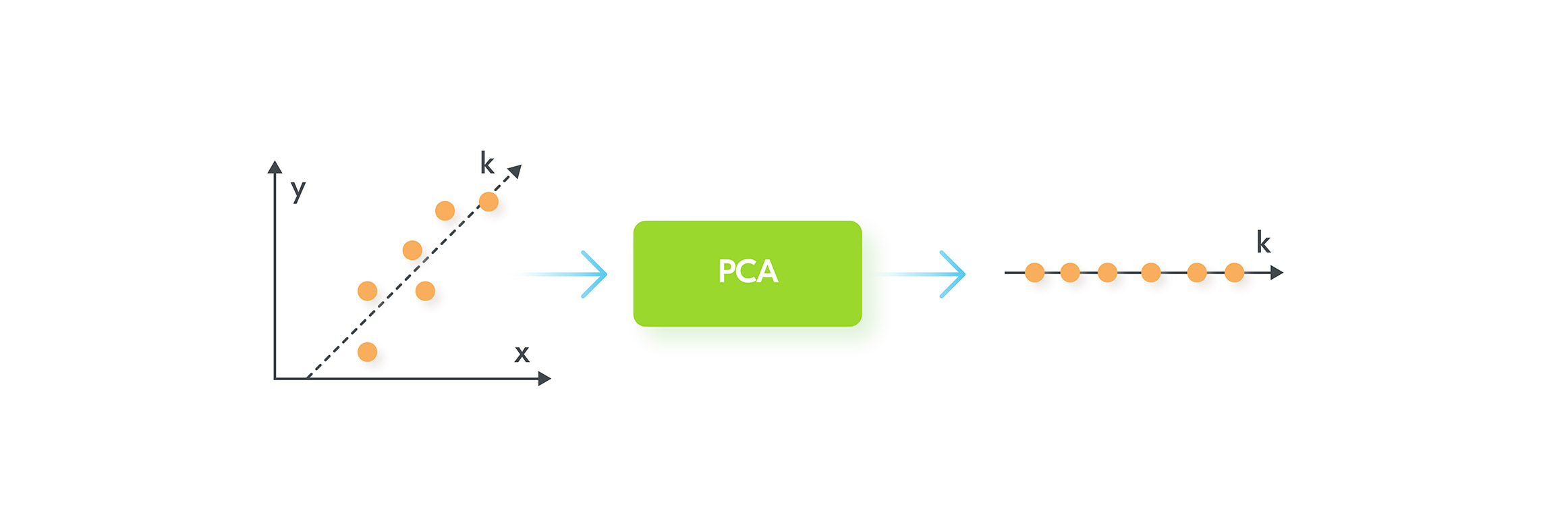
Reinforcement Machine Learning
AI is often used as a buzzword in places where the speaker really means ML. But Reinforcement Learning (RL) is an exception. Because, most of the time RL deals exactly with AI goals – creating an agent which can make efficient actions in a provided environment. RL algorithms use reward as feedback to taken actions, and try to maximize it.The rise of Reinforcement ML’s popularity began after the famous Go game match between Google’s AI – AlphaGo and human champion – Lee Sedol. AlphaGo was created using Reinforcement ML. Even the first version of the AI was a serious challenge for any human player. The next edition – AlphaZero reached a level of complexity unachievable for humans. The distinctive feature of AlphaZero is that it learned to play with itself, rather than use human parties for supervised learning.Currently, the main research in RL is focused on building AI for different classic video games and making a machine figure out everything by itself. To put that another way, AI at first does not know anything about the game environment and knows only a few actions. It tries to apply those actions and obtain feedback from the game. This allows it to improve itself via the mechanism of reward/penalty. Each good action rewards the AI, each unsuccessful action penalizes it.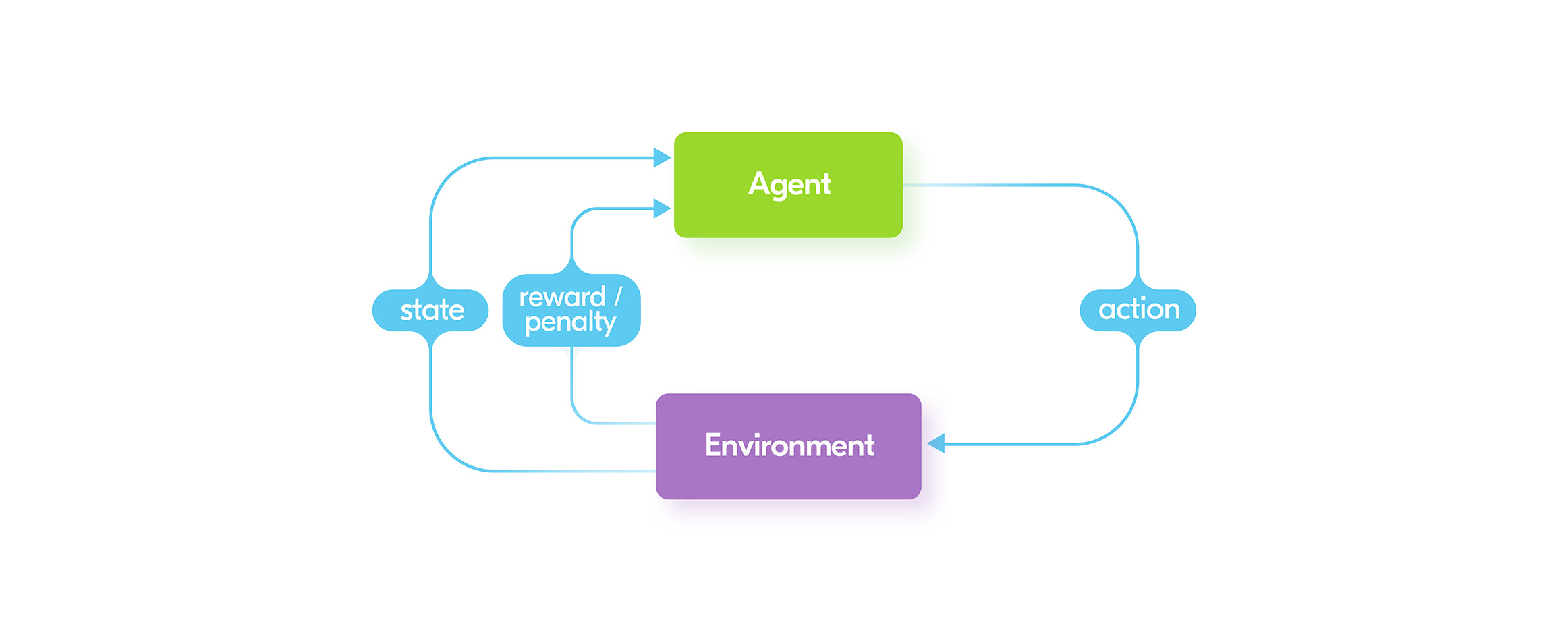