The world of finance deals with statistical data and quantitative figures that make it a perfect area for machine learning (ML).
This engineering science has found its use in different areas such as healthcare, travel, media, and retail.
At the same time, financial services also benefit a lot from deploying “thinking” computer systems. As a result, financial institutions achieve a fast, reliable, and accurate solution to cope with an unlimited range of tasks associated with data analysis, processing, and interpretation.
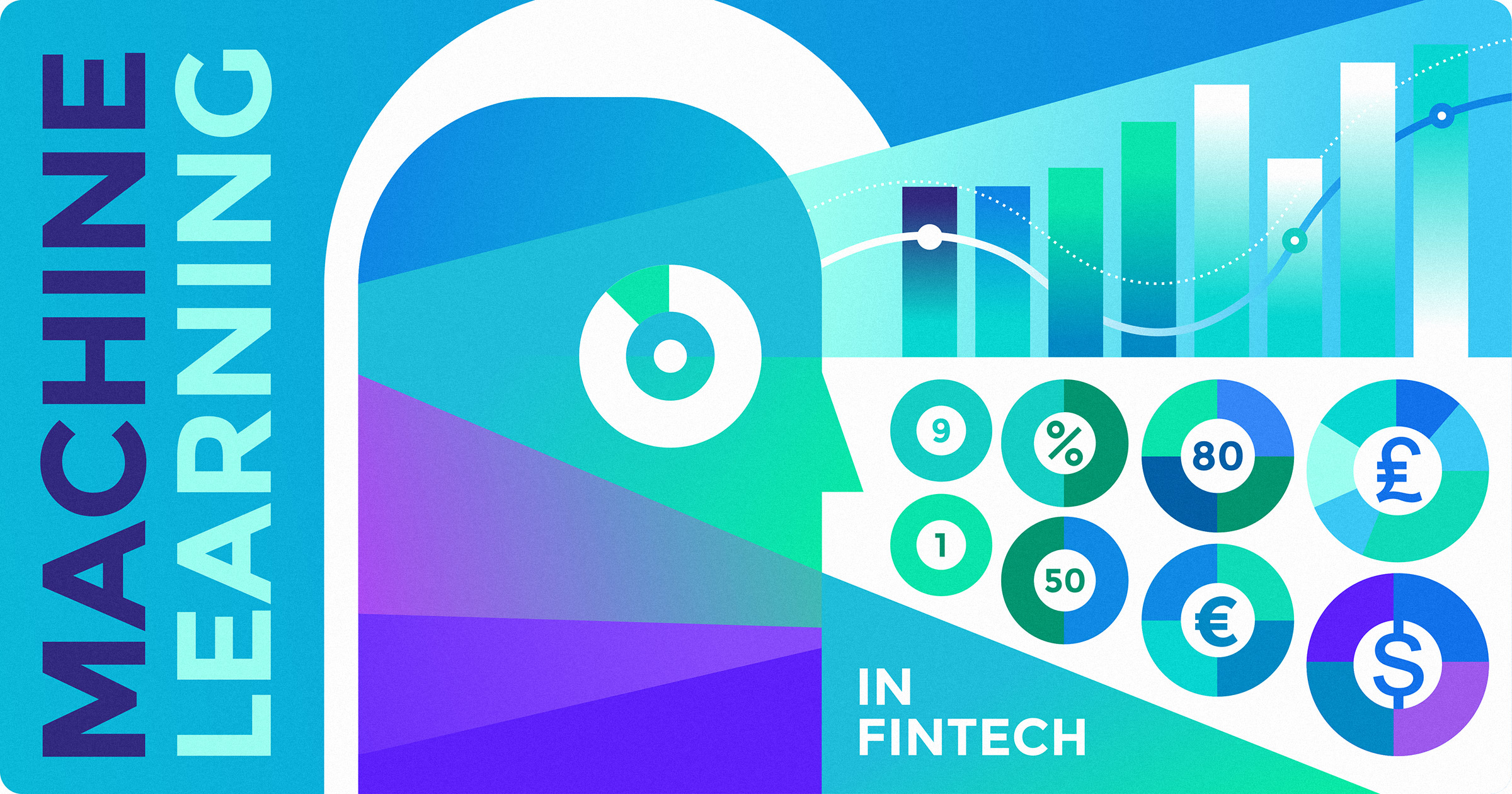
The money world requires fast and sometimes immediate actions. Therefore, speed is vital for a company to become a competent player in the fintech market. Machine learning algorithms can accelerate data processing and provide a solution for managing a vast amount of data.
Another benefit of using artificial intelligence in finance is that it’s unbiased. You’ll find a computer with bad intentions which means you can trust machine learning algorithms for trading securities and closing multi-million deals.
Unlike humans, who are to make errors when dealing with repetitive tasks, well-tailored algorithms provide a lower risk of mistakes. Accuracy is the third-important major benefit of machine learning. In this review, we are going to see how financial services can benefit from implementing ML and look at the possible applications in fintech.
Application of machine learning in finance
Some common applications of machine learning include voice recognition, email spam filtering, text processing, search request analysis, etc. In fintech machine learning algorithms are used in chatbots, search engines, analytical tools, and versatile mobile banking apps. In fact, a financial ecosystem is a perfect area for AI implementation. The number of companies using machine learning keeps growing because machine learning is not a trend, but a robust optimization solution. The following application cases are the proof of this statement.
Digital advisors
So-called robo-advisors perform the function of financial experts, providing advice to customers — only without any human intervention. This term is used to define algorithms tailored to adjust financial portfolios or an investment plan according to user’s risks and purposes.
According to FINRA, there are several activities incorporated by these algorithms: allocation of assets, customer profiling, portfolio adjustment/rebalancing, trading,and tax-loss collection. The main benefit observed with robo-advisors is the lack of the emotional element associated with investment account management. In addition, this electronic tool is cheaper than traditional investment consultancy that makes it more attractive to less-affluent clients.
Today, we can observe a substantial growth of the number of machine learning solutions to apply to trading needs. One of the differences between traditional algorithmic systems and machine learning is that the latter is able to adapt to immediate changes according to the accumulated experience. There are different algorithm-optimization strategies like deep learning, neural networks, linear regressions and much more to be implemented in the state-of-the-art trading tool. Sentiment analysis (described below) is another secret weapon of machine learning in algorithmic trading, which is highly estimated by numerous hedge funds.
Trading companies have hundreds of ML algorithms at their disposal and can use each of them for specific work. The range of purposes includes trade predictions, determination of strategy parameters, analysis of market behavior, etc. For example, to build a model of decisions requires a decision tree algorithm; regression algorithms can be applied to solve regression problems and shape relationship between variables. Quants opt for ML and AI systems as a helpful tool for versatile purposes involved in estimation of investments and risks.
Security and fraud detection
Unfortunately, fraudulent activities develop alongside with fintech companies. With that in mind, ensuring the data security of clients is an essential goal for any financial services provider. Paradoxical though it might sound, the advent of contemporary mobile banking apps and related software gives rise to new forms of fraudulence. As a result, traditional security systems, which are based on a set of fixed rules and policies, give way to fraud detection machine learning solutions that deploy risk factors experience to adapt to new potential threats. Security Orchestration, Automation and Response (SOAR) platforms further complement these efforts by integrating multiple security tools, automating responses, and coordinating actions across different systems to detect and mitigate threats more efficiently.
Today cybersecurity measures like recognition of different biometric data are deployed along with fraud prevention systems. Alongside biometric data recognition, cybersecurity teams use fraud prevention tools including carrier lookup to verify phone numbers, CAPTCHA systems to block bots, and geolocation tracking to identify suspicious login attempts. Sift Science is a good example of a company that deploys the capabilities of machine learning in quantitative finance to protect business from possible losses associated with fraudulence like account takeover, content abuse, and payment fraud.
Underwriting
Underwriting in banking, insurance or securities is the perfect niche for financial technology based on machine learning. As a rule, large underwriters maintain whole departments and numerous staff to cope with analysis and evaluation of potential risks to insure applicants and their assets. However, a customized algorithm can handle tons of routine tasks related to processing client’s personal data, as well as credit reputation, and insurance history.
Machine learning in the insurance industry is becoming more popular because corporations can afford to develop advanced algorithms for processing massive volumes of data. Unfortunately, smaller companies have limited financial resources, and joining the trend still seems a pipe dream.
Customer service
Automating customer service can have negative consequences, since not all clients are happy to listen to a recorded voice and follow speech prompts. However, artificial intelligence used for customer services provides a completely different experience. Conversational interfaces and digital assistants are able to direct a client to the proper executive or department, as well as answer uncommon questions regarding the balance status or payment report for a specific period in a human way. An AI voice agent can also manage routine inquiries, providing instant responses while freeing up human staff for more complex tasks. And the most incredible thing is that no human interaction is needed. The AI technology is able to recognize patterns and behavior, as well as understand and respond to unique requests.
Sentiment analysis
This term can be explained as a thorough analysis of information to determine the emotional tone and understand the opinion behind a certain subject. Sentiment analysis has a broad application range, from building an understanding of the social sentiment of the product/service to making predictions regarding the estimation of trades and stock prices.
With capabilities provided by ML algorithms, the quality and accuracy of sentiment analysis considerably increases. Naturally, we are not claiming that machine learning can compare to the so-called human sixth sense (only humans have intuition), but the technology offers exciting possibilities.
Categories of fintech solutions where machine learning is used the most
Since fintech is defined as the use of technological concepts for financial transactions, its ecosystem covers a wide range of categories including payments, insurance, investment, lending, etc. The following options are the most promising and investment-attractive segments for implementing machine learning.
Payments & remittances
Innovative payments are an integral part of e-commerce development and globalization’s growth. The number of digital payments and money transfers made with various electronic payment methods (e-wallets, payment cards, mobile apps and others) all over the globe runs into billions. With machine learning, the payments & remittances segment can process a pile of transactions in a fast and secure way.
For example, the online payment service Klarna uses ML techniques to build the fraud-preventing models that can distinguish true and false user’s transactions. New and existing merchants are also analyzed to minimize possible risk and optimize performance.
Another representative of this category, TransferWise, is a peer-to-peer money transfer service, which uses AI benefits for declarative infrastructure management and preventing financial crimes.
Lending & financing
P2P lending excludes any necessity in banks or credit companies, because ordinary people can perform direct lending transactions without any intermediary. With Kabbage, an online P2P lending service, you can both lend and borrow your money using your laptop or smartphone. ML algorithms implemented in the app analyze public profile data and other information to determine an applicant’s eligibility. In addition, portfolio analysis and credit risk models are also based on AI advances.
Retail banking
Electronic banking is probably the most widespread application of advanced innovations in this category. At the same time, banks leverage machine learning techniques for numerous purposes including customer experience, operational efficiency, budget optimization, privacy protection, etc.
Ally is one of the first US-based banks to look towards machine learning as an essential tool for automating transactions associated with banking management and securing its customers. At the same time, fraud protection is not the only use of ML. Mobile banking UX has become more refined, since the technology predicts what a client wants to do.
JPMorgan Chase, a leading global financial services firm, applied the ML technology to design a COiN platform for analyzing specific data and documents. Several seconds of automated tasks replace thousands of hours spent on manual analysis.
Insurance
The insurance industry is characterized by tons of data required for underwriting and decision-making processes. On that account, the industry is deemed one of the most attractive fields for machine learning. Today, three applications including digital assistants, insurance market analytics and driver performance monitoring are taking the lead.
Liberty Mutual, an American diversified global insurer, applies the AI benefits for developing a new damage-assessment app. It provides real-time analysis of vehicle damage using a mobile device camera. The app’s ML model is based on multiple vehicle-crash images to estimate a repair cost.
Insurtech is not completely dominated by giant corporations. Numerous state-of-the-art startups regularly add uncommon features. For example, an Italian virtual insurance agent, Neosurance, boasts interaction between a machine learning system and IoT to analyze and learn information about a client. As a result, a single swipe is enough to get insured using your smartphone.
Blockchain & cryptocurrencies
The blockchain is rightly deemed the most promising technology in the coming years. The initial use for cryptocurrencies has spread across miscellaneous applications in different industries and businesses. With blockchain, fintech obtains a huge potential to grow and become more efficient. Machine learning can be considered a booster to the said technology since their convergence can ensure an incredibly fast analysis of enormous volumes of data. Moreover, security also improves if the chain is governed by artificial intelligence.
For example, a well-known service dealing with cryptocurrency transactions, Coinbase, opted for a new ML-based identity verification system when it had faced troubles with a slow user verification. Now, buying/selling digital currency like Ethereum and Bitcoin via the service is much more secure than before.
How does machine learning in financial services work?
We have already discussed application and classification of AI and ML, and found answers to the question “How is machine learning used?” However, the actual working principle of the technology arouses heightened interest.
Any ML system consists of three parts – model, parameter, and learner. Model is the inception, which will be the basis for further prediction. However, to make calculations, the model requires parameters to be set. All this is wrapped in a mathematical equation to get an initial input. For example, a certain time spent on jogging causes a specific calories reduction:
- 1 hour = 1100 kcal
- 30 minutes = 550 kcal
- 15 minutes = 225 kcal
and so on
The model is set but the real life input can be different that triggers the learning part. The learner in the ML system uses a particular algorithm based on the set parameters and the model to build predictions. It looks at deviations and adjusts initial assumptions:
- 1 hour = 1200 kcal
- 30 minutes = 600 kcal
- 15 minutes = 300 kcal
and so on
The new forecast is shaped.
Machine learning includes three branches – reinforcement, supervised, and unsupervised learning. The first one stipulates learning through training, while supervised/unsupervised options are more complex. In supervised learning, the program makes forecasts using a trial-and-error approach according to the pre-set input and output. In unsupervised learning, the output is unknown, and the program’s task is to find patterns within the data.
To sum up, an algorithm underlying the machine learning system has to find a relationship between output and input data.
Artificial intelligence’s influence on the future of fintech
Today, the effect of technological innovations is clearly visible in banking, insurance, crowdfunding and other fields. And what to expect next? The future of algorithmic trading and other applications in the financial industry is approaching the humanization of computers. Data processing is shifting to a more advanced level where digital assistants will become full-fledged labor resources and traditional monetary services will gradually fade. We’re on the threshold of a new era of machine learning financial services.
Railsware’s machine learning experience
We at Railsware use the benefits of machine learning techniques in our work. At the moment, supervised (linear and logistic regression, support vector machines) and unsupervised (anomaly detection, k-means) learning are basic algorithms we use to build predictions, make data classifications, recommendations, and collective filtering. However, we’re looking into supervised learning with neural networks and more complex applications like image/pattern recognition, time series prediction, function approximation, and clustering.
One of the Railsware’s fintech projects where we implemented machine learning algorithms is Quorso, a SaaS solution that helps companies improve their profits by analysing their financial data. This B2B platform is packed with innovative technologies including ML for forecasting. The implemented recommendation system provides suggestions based on the profit and loss analysis.
Conclusion
The future is already upon us, and implementing machine learning in financial field bears witness to this statement. And Railsware is right in the thick of it, contributing to the contemporary industrial revolution by developing numerous fintech solutions. You can and should keep up with the times, and streamline the state-of-the-art fintech innovations.